Notes on the Book "Effective Pandas"
The book I introduced in the last post has turned out to be so fantastic that I have decided to make notes of it. This is a live document; I started from Chapter 21 and am going on from there. I will return to the earlier chapters if I still have time and power. The author recently appeared in Real Python Podcast: Becoming More Effective at Manipulating Data with Pandas and talked about the book.
- 1. Introduction
- 2. Installation
- 3. Data Structures
- 4. Seris Introduction
- 5. Series Deep Dive
- 6. Operators (& Dunder Methods)
- 7. Aggregate Methods
- 8. Conversion Methods
- 9. Manipulation Methods
- 10. Indexing Operations
- 11. String Manipulation
- 12. Date and Time Manipulation
- 13. Plotting with a Series
- 14. Dates in the Index
- 15. Categorical Manipulation
- 16. Dataframes
- 17. Similarities with Series and DataFrame
- 18. Math Methods in DataFrames
- 19. Looping and Aggregation
- 20. Columns Types, .assign, and Memory Usage
- 21. Creating and Updating Columns
- 22. Dealing with Missing and Duplicated Data
- 23. Sorting Columns and Indexes
- 24. Filtering and Indexing Operations
- 25. Plotting with Dataframes
- 26. Reshaping Dataframes with Dummies
- 27. Reshaping by Pivoting and Grouping
- 28. More Aggregations
- 29. Cross-tabulation Deep Dive
- 30. Melting, Transposing, and Stacking Data
- 31. Working with Time Series
- 32. Joining Dataframes
- 33. Exporting Data
- 34. Styling Dataframes
- 35. Debugging Pandas
- 36. Summary
Introduction
Installation
Data Structures
Seris Introduction
Series Deep Dive
Operators (& Dunder Methods)
Aggregate Methods
Conversion Methods
Manipulation Methods
Indexing Operations
String Manipulation
Date and Time Manipulation
Plotting with a Series
Dates in the Index
Categorical Manipulation
Dataframes
Similarities with Series
and DataFrame
Math Methods in DataFrames
Looping and Aggregation
Columns Types, .assign
, and Memory Usage
Creating and Updating Columns
p. 179. I think the 8th row of the code .replace({'Yes' : True, 'No' : False, np.nan : False})
could be made more concise like .eq('Yes')
.
Dealing with Missing and Duplicated Data
Sorting Columns and Indexes
p. 194. Ths is a code in the book to sort a list of American presidents according to ther last names.
>>> (pres
.sort_values(
by='President',
key=lambda name_ser: name_ser
.str.split()
.apply(lambda val: val[-1]))
)
Seq President Party ...
2 2 John Adams Federalist ...
6 6 John Quincy Adams Democratic-Republican ...
An apply method and a second lambda function are necessary as shown in the above example; you cannot split a series and access the last string by indexing [-1] in a single lambda function.
>>> (pres
.sort_values(
by='President',
key=lambda name_ser: name_ser
.str.split()[-1])
)
ValueError
....
Filtering and Indexing Operations
pp. 199-200. I don't see the point in first setting the index for the 'President' column and then resetting it, instead of directly resetting it.
(pres
.set_index('President')
.reset_index()
)
p. 201. Note. Now I know why '&' in df.loc[...]
sometimes requires parentheses next to it! The book says that "the & operator has higher precedence than >=" and the likes and that as a result, Pandas interprets the double condition pres.Average_rank < 10 & pres.Party == 'Republican'
as pres.Average_rank <
(10 & pres.Party) == 'Republican'
while you meant (pres.Average_rank < 10) & (pres.Party == 'Republican')
, for example. The author recommends that "you should always put parentheses around multiple conditions in index operations." I will keep that in mind.
p. 201. The use of the prefix '@' in the query method was totally new to me. It looks to incorporate variables defined outside into the query string.
>>> Roosevelts = ['Theodore Roosevelt', 'Franklin D. Roosevelt']
>>> (pres
.query('President.isin(@Roosevelts)')
[['President','Party']]
)
President Party
25 Theodore Roosevelt Republican
31 Franklin D. Roosevelt Democratic
Plotting with Dataframes
p. 224
(pres
.set_index('President')
.loc[:,'Background':'Average_rank']
.iloc[:9] # This is synonymous with .head(9)
.T
)
Reshaping Dataframes with Dummies
pp. 231-2. The row .filter( like = r'jb.role.*t' )
in the codes doesn't seem to make sense. I suppose that it should be .filter( like = 'jb.role')
. The r prefix does no harm while it is unnecessary.
I don't believe I have grasped this chapter well.
Reshaping by Pivoting and Grouping
p.238. jb2 defined in p. 181 does not work here; the age columns gives a TypeError seemingly because its dtype is Int64. Switching to a float resolves the issue.
>>> (jb2
# .astype({'age' : float}) This line is necessary
.pivot_table(index='country_live',columns='employment_status',values='age',aggfunc='mean')
)
TypeError: Int64
pd.crosstab(
index=jb2.country_live,
columns=jb2.employment_status,
values=jb2
.age
.astype(float), # So is this line.
aggfunc="mean",
)
Interestingly, the groupby method doesn't require a conversion of the age column into float numbers.
p. 248. How useful pivot_table is! The order of min and max is somehow reversed.
>>> (jb2
.pivot_table(
index='country_live',
values=['age', 'company_size'],
aggfunc=({'age': ('min', 'max'), 'company_size': 'mean'})
)
)
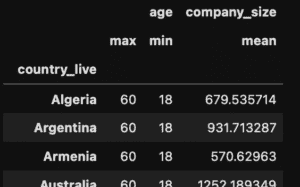
p. 249. This inconsistency of Pandas is annoying; mean
requires quotation marks while min
and max
do with or without them.
(jb2
.groupby('country_live')
.agg(age_min=('age',min),
age_max=('age','max'),
team_size_mean=('team_size','mean')
)
)
pp. 252-253. It's wonderful pivot_table
and groupby
don't only accept values but functions.
def even_grouper(idx):
return 'not multiiples of 3' if idx % 3 else 'multiples of 3'
jb2.pivot_table(index=even_grouper,aggfunc='size')
More Aggregations
The .transform method, which is new to me, assigns aggregated information of groups such as size and mean to each member.
#28.4 Excercises
#1
(jb2
.assign(pvmsum=(jb2
.groupby('python3_version_most')
.age
.transform('sum')
)
)
)
#2
(jb2
.groupby('country_live')
.filter(lambda g:g.age.size>=3)
)
2 thoughts on “Notes on the Book "Effective Pandas"”